Modern professional astronomers aren’t much like astronomers of old. They don’t spend every suitable evening with their eyes glued to a telescope’s eyepiece. You might be more likely to find them in front of a super-computer, working with AI and deep learning methods.
One group of researchers employed those methods to find a whole new collection of stars in the Milky Way; a group of stars which weren’t born here.
The group of stars is called Nyx. Nyx is a vast stream of stars that’s near the Sun, near being a relative term of course. The team of researchers say that Nyx is the stretched out remains of a dwarf galaxy or globular cluster that merged with the Milky Way.
The new study presenting their results is titled “Evidence for a vast prograde stellar stream in the solar vicinity.” The lead author is Lina Necib, a postdoctoral scholar in theoretical physics at Caltech. The paper is published in the journal Nature Astronomy.
One of the interesting things about this discovery is how the researchers found it. Nyx wasn’t spotted with a telescope, it was found by applying deep-learning methods to data from Gaia, the ESA’s space observatory that’s charting a 3D map of the Milky Way. This discovery is a reflection of how many of our discoveries are made these days. Instead of probing the skies with telescopes directly, automated sky surveys gather enormous amounts of data that can then be studied itself.
The study identified about 200 to 250 stars that makeup Nyx. Finding these remnants of other smaller galaxies shouldn’t be surprising; this is how galaxies grow. But non-scientists might be surprised by how it was found.
“We’re at the beginning stages of being able to really understand the formation of the Milky way.”
Lina Necib, Lead Author, Theoretical Physicist, Caltech
Gai is creating a 3D map of the Milky Way, but not by charting each individual star; there are over 200 billion of them. Gaia does it by sampling about one billion of the galaxy’s stars, and extrapolating from them. Another research effort, called Feedback In Realistic Environments (FIRE), creates massive cosmological simulations that seek to improve the predictive nature of our galaxy formation models, among other things.
The team of researchers behind this study used both Gaia and FIRE to discover Nyx. They combined the data from both and subjected it to what are called “deep learning methods.” Deep learning methods are a complex topic, and not easily explained. Basically, very basically, it’s a method of machine learning that uses things like artificial neural networks. If you want to know more, head to Cambridge Mass. and hang around the MIT campus. Advanced modelling shows that’s your best bet.
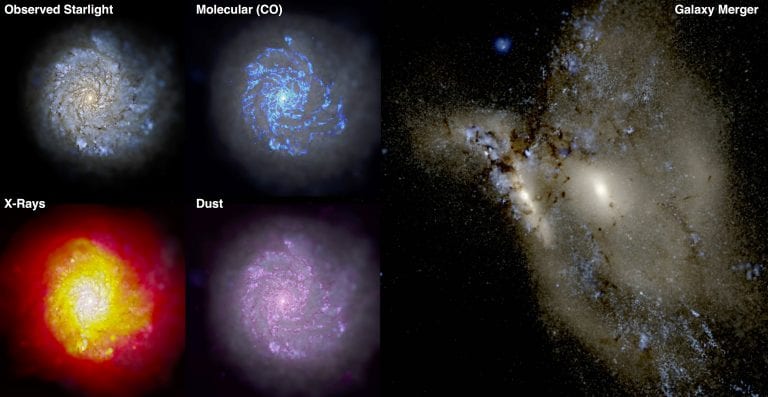
“It’s the largest kinematic study to date. The observatory provides the motions of one billion stars,” Necib said of Gaia in a press release. “A subset of it, seven million stars, have 3D velocities, which means that we can know exactly where a star is and its motion. We’ve gone from very small datasets to doing massive analyses that we couldn’t do before to understand the structure of the Milky Way.”
We know that galaxies like the Milky Way grow large through mergers. Not necessarily the titanic mergers like the one that’ll take place a billion years from now, between the Milky Way and Andromeda. More like a series of smaller mergers, like the one in this study. The Milky Way has over 50 small satellite galaxies, and mergers between it and these types of smaller ones are likely an incremental process in the growth of galaxies.

Scientists are quite certain about mergers fuelling galactic growth, but evidence of it has been hard to find in the Milky Way. Things seems quiet in our galaxy—too quiet, as far as some researchers are concerned.
“Galaxies form by swallowing other galaxies,” Necib said. “We’ve assumed that the Milky Way had a quiet merger history, and for a while it was concerning how quiet it was because our simulations show a lot of mergers. Now, with access to a lot of smaller structures, we understand it wasn’t as quiet as it seemed. It’s very powerful to have all these tools, data and simulations. All of them have to be used at once to disentangle this problem. We’re at the beginning stages of being able to really understand the formation of the Milky way.”
As the press release for this study makes clear, the Gaia map presents its own challenges. All of its data is a treasure trove that was simply out of the reach of previous generations of researchers. But how can you handle all that data? How can researchers parse through it all to find the connections and patterns? That’s where powerful computers and machine learning come in.
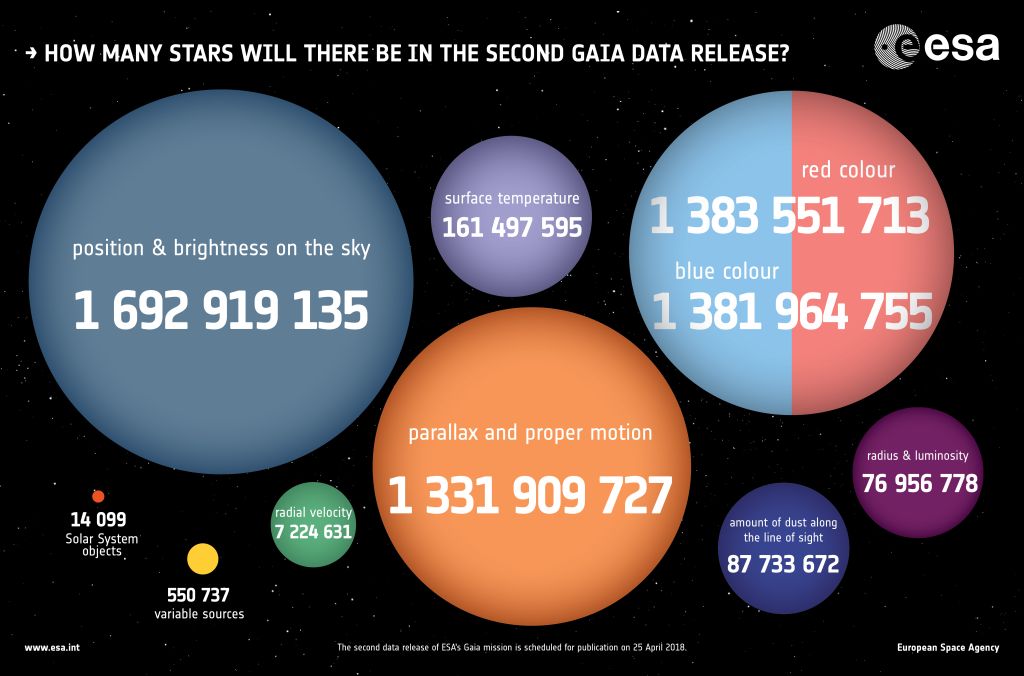
“Before, astronomers had to do a lot of looking and plotting, and maybe use some clustering algorithms. But that’s not really possible anymore,” Necib explained. “We can’t stare at seven million stars and figure out what they’re doing. What we did in this series of projects was use the Gaia mock catalogues.”
Before we explain what the Gaia mock catalogues are, there’s something you need to know about simulations and machine learning and related tools. As powerful as they are, they have a flaw. That’s not surprising; everything does.
The danger in this type of work is similar to what haunts all computers, and it was formulated quite succintly in the early days of computers: Garbage In, Garbage Out (GIGO). What that means is that no matter how powerful a computer is, it can’t produce results if its inputs are garbage. In a more nuanced way, that’s the pitfall for supercomputers and machine learning, and for simulations like FIRE.
One of the study collaborators is Bryan Ostdiek (formerly at University of Oregon, and now at Harvard University), who had previously been involved in the Large Hadron Collider (LHC) project. Ostdiek has vital experience dealing with massive datasets and machine learning at the LHC.
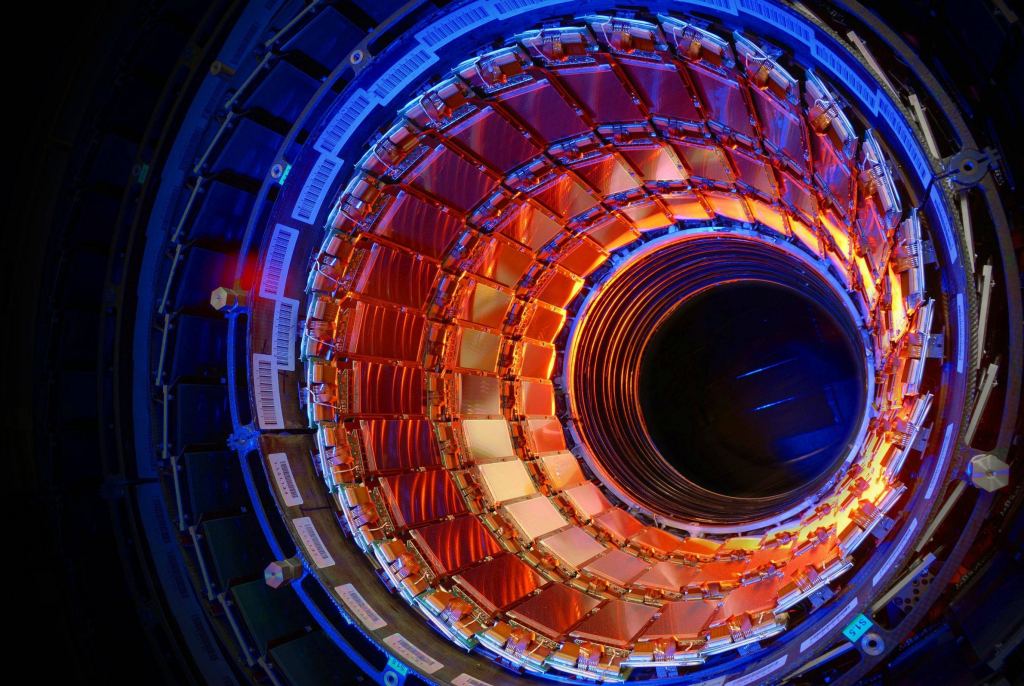
“At the LHC, we have incredible simulations, but we worry that machines trained on them may learn the simulation and not real physics,” Ostdiek said. “In a similar way, the FIRE galaxies provide a wonderful environment to train our models, but they are not the Milky Way. We had to learn not only what could help us identify the interesting stars in simulation, but also how to get this to generalize to our real galaxy.”
The famous Polish-American scientist and philosopher Alfred Korzbski summed up the problem when he said, “The map is not the territory.”
“We needed to make sure that we’re not learning artificial things about the simulation, but really what’s going on in the data.”
Lina Necib, Lead Author, Theoretical Physicist, Caltech
The team started with FIRE simulations of galaxies. They then grouped the stars in those galaxies into two types: stars born in the simulated host galaxy, or stars that came from mergers. The differences between the two can be subtle, but they’re there. Those labels were then used to train the deep learning models, which the team then tested on other FIRE simulations.
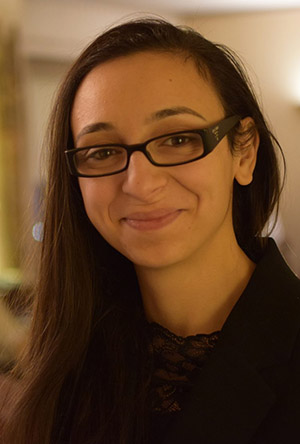
The result of all of that is the Gaia mock catalogues. They then took the catalogues and the deep learning model and applied it to the real Gaia data. In essence, they asked a simple question: “‘Based on what you’ve learned, can you label if the stars were accreted or not?'” Necib said. The model then ranked each star according to how confident it was in the label, either home-grown star or merged star.
If this sounds convoluted, and fraught with potential errors, it is. But it’s also powerful, and for teams with the right expertise, and who put in a lot of hard work, it can be powerful.
The entirety of the method the researchers are using is called “transfer learning.” And Necib knows what the dangers are. “We needed to make sure that we’re not learning artificial things about the simulation, but really what’s going on in the data,” Necib said. “For that, we had to give it a little bit of help and tell it to reweigh certain known elements to give it a bit of an anchor.”
Results like these can be tested. They can set the deep learning model loose on the actual Milky Way, and see if it can identify known features. The team tested it with what’s called the Gaia Sausage.
The Gaia Sausage is the tell-tale form of a dwarf galaxy that merged with the Milky Way between 8 to 11 billion years ago. The population of stars in the Gaia Sausage occupy distinct velocity space, in a characteristic sausage shape of elongated orbits.
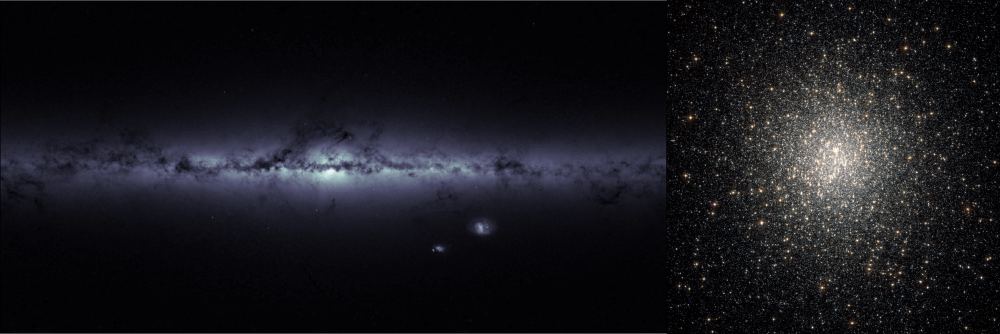
“It has a very specific signature,” she explained. “If the neural network worked the way it’s supposed to, we should see this huge structure that we already know is there.”
The test was successful. Not only did the neural network find the Gaia Sausage, it found something else: Nyx. A stream of stars that was rotating with the Milky Way, but was also moving as a group toward the galaxy’s center.
“Your first instinct is that you have a bug.”
Lina Necib, Lead Author, Theoretical Physicist, Caltech
When she spotted the stellar stream of stars, Necib thought it might be a bug. “Your first instinct is that you have a bug,” Necib recounted. “And you’re like, ‘Oh no!’ So, I didn’t tell any of my collaborators for three weeks. Then I started realizing it’s not a bug, it’s actually real and it’s new.”
The next question, since this is science and scientists are thorough, was to look through the scientific literature to see if this stellar stream of stars had been identified before.
“Everything about this project is computationally very intensive and would not be able to happen without large-scale computing.”
Lina Necib, Lead Author, Theoretical Physicist, Caltech
“You start going through the literature, making sure that nobody has seen it and luckily for me, nobody had. So I got to name it, which is the most exciting thing in astrophysics,” Necib said. “I called it Nyx, the Greek goddess of the night. This particular structure is very interesting because it would have been very difficult to see without machine learning.”
When the European Space Agency launched Gaia in 2013, they knew it would produce an enormous amount of data. Researchers knew at the time that they would have to develop better methods to take all of that data and make sense of it. And this study is a great example of how it’s working out.
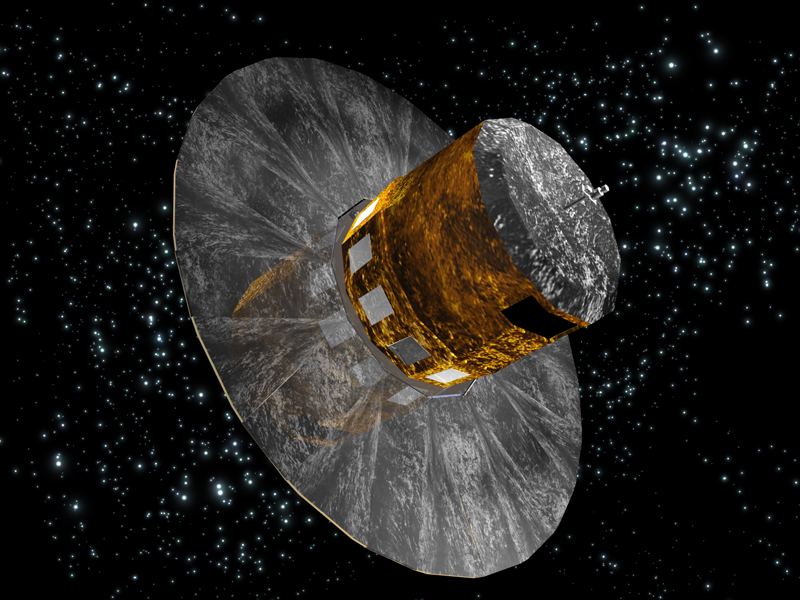
“When the Gaia mission started, astronomers knew it was one of the largest datasets that they were going to get, with lots to be excited about,” Necib said. “But we needed to evolve our techniques to adapt to the dataset. If we didn’t change or update our methods, we’d be missing out on physics that are in our dataset.”
“Everything about this project is computationally very intensive and would not be able to happen without large-scale computing,” Necib said.
This isn’t the end, though. Next will come spectroscopic studies of Nyx’s stars. That’ll help researchers understand where Nyx came from. It should help them figure out if Nyx is definitely the remnants of a dwarf galaxy. There’s also a possibility that Nyx isn’t a stream of stars from another galaxy, but a perturbation in the Milky Way’s disk with a different cause.
“Follow-up spectroscopic studies will play a crucial role in establishing the origin of the Nyx stream by providing a larger set of chemical abundances,” the authors write in the conclusion of their paper. “This will help either confirm the kinematic arguments above that suggest that Nyx is the remnant of a dwarf galaxy, or that it is a perturbation of the Milky Way disk.”
More:
- Press Release: NEW COLLECTION OF STARS, NOT BORN IN OUR GALAXY, DISCOVERED IN MILKY WAY
- Research Paper: Evidence for a vast prograde stellar stream in the solar vicinity
- Universe Today: Gaia Spots an Enormous Ghost Galaxy Right Next Door that’s Being Dismantled by the Milky Way